How AI is used to detect lateral movement: Use case
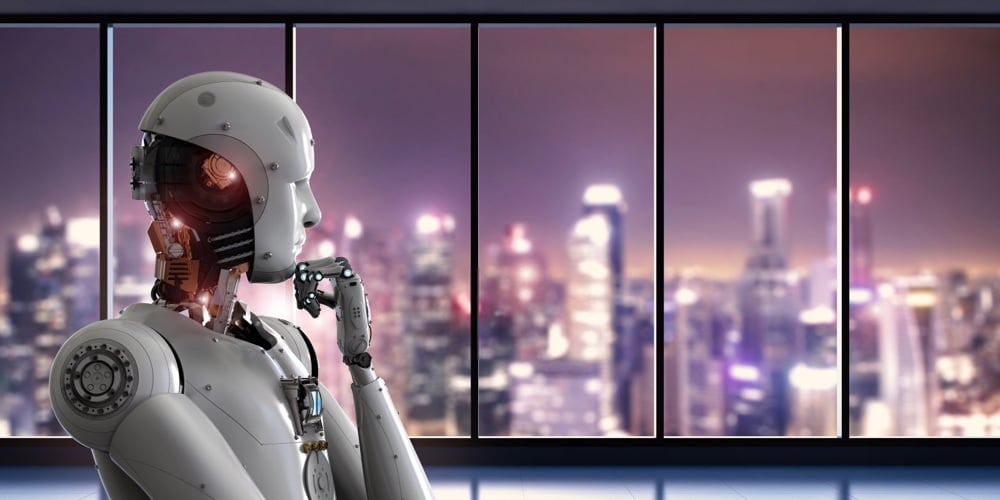
Adlumin recently flagged lateral movement incidents on a customer’s network. The detection was achieved via an AI algorithm designed to aggregate suspicious incidents until they collectively project a high-fidelity threat signal. This prevented further compromise of valuable resources, and Adlumin detection response teams advised the client on remedial action.
Lateral movement
When a cybercriminal first gains access to a network, they often move laterally to different machines until they find what they’re looking for. This movement can usually be traced using Windows access-event logs. However, detecting this behavior automatically amongst the enormous corpus of access events can be extremely difficult, especially when it involves privileged users with network-wide access. This is where Adlumin AI comes into play.
Adlumin AI
A machine-learned algorithm assigns an anomaly score to each successful logon event that occurs on an Adlumin customer’s machine. Anomalous logon events are then aggregated to form access graphs, which are subsequently assessed for attack signatures. For example, many anomalous logon events from a single user on a single machine may indicate that an attacker is attempting to gain access to network share drives or performing scanning-like behavior to gain a foothold elsewhere on the network. Below is an example of a penetration test detected on a customer network where the user attempted to access 15 separate machines.
continue reading »