Machine learning can reduce fraud risk and improve profitability
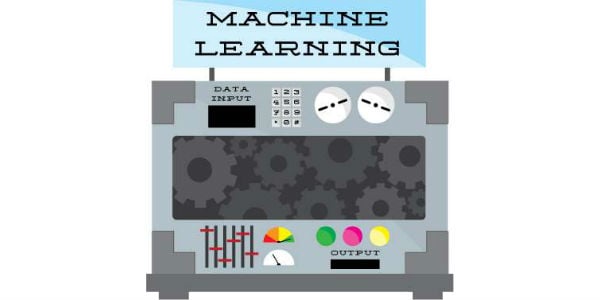
by. Tom Donlea
The digitization of financial transactions and growth of mobile banking have tremendously increased the amount of information banks need to evaluate for fraud. This presents new challenges to Chief Risk Officers who use human analysis of these large data sets to identify new risk threats. The answer lies in using automated analysis of data, or machine learning, to keep pace with fraudsters and maintain an affordable process.
Machine learning, a branch of artificial intelligence, simply means that the computer can learn from data rather than being explicitly told (programmed) what to do. For example, a machine learning system could learn to distinguish between suspicious account behavior and normal customer actions.Similarly, machine learning can ascertain patterns that signal fraud in account sign-ups to prevent fraudsters from entering the system.
The Evolution of Risk Management
Most lenders have built home-grown systems that use business rules to manage their fraud detection processes. These hand-crafted rules are framed as “if-then” statements. An example would be: “if several transactions are made within a short amount of time in a different state, then send the account to manual review.” These rules have been built and refined with decades of experience analyzing fraud data. Many of the rules are set up to provide additional analysis for unusual account behavior or suspect information at the point of account sign-up.
As the amount of data being produced and the complexity of analyzing has grown to unprecedented levels, the manual process of building and maintaining business rules is becoming more expensive, more time intensive, and less predictive.
continue reading »