Why transaction data cleansing is an operational imperative
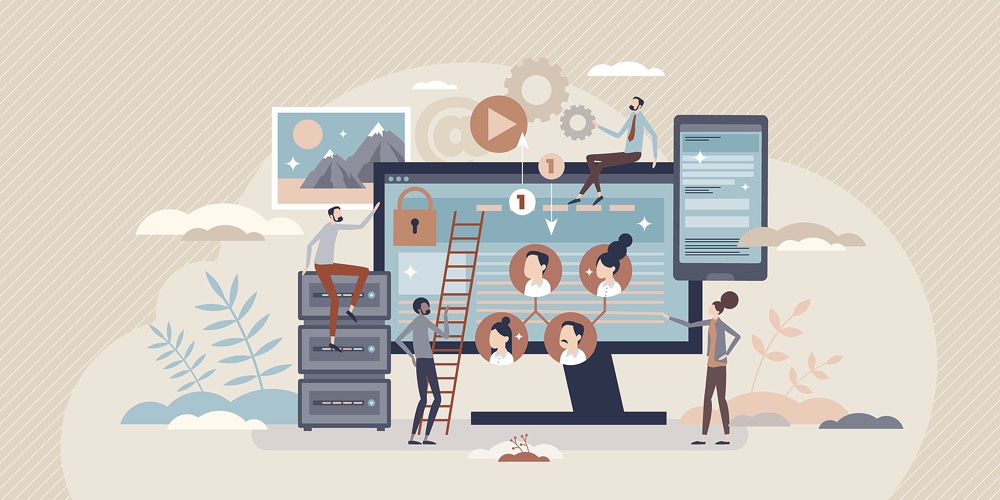
An unfortunate rule of thumb is that a data scientist spends 80% of the time cleaning and prepping data and only 20% of the time actually analyzing data for insights. Transaction data is a rich source of information about account holders, but it is notoriously cryptic and non-standardized.
Cryptic merchant names on transactions seem deliberately vague, don’t they? Who would have guessed that “DOCTORSASSOCINC” is actually Subway Restaurants? With that kind of “clarity” it’s easy to see that accurately performing the identification and matching of millions or even billions of raw transaction strings to the right merchants, at scale, requires significant resources and expertise.
Although it holds enormous value, unless it is cleansed and categorized, transaction data is unusable for analysis. Understanding that data allows a credit union to better analyze member behavior and spending patterns, allows them to develop deeper relationships with members. Without an understanding of members, they can’t reach out to them with relevant and timely offers and information, and grow their business in the process.
The ideal solution to this challenge is through transaction data cleansing. Credit unions of all sizes can seek outside assistance from financial data analysis experts who excel at performing automated, accurate, daily data cleansing.
Transaction Data Cleansing Is Necessary For Transforming Raw Data Into Insights
- Enhanced UX and Optimizing Resources
Give members a 5-star online banking experience, avoid transaction disputes by providing better online banking statements. Even reduce IT hours, lag time and quality issues associated with cleansing data in-house.
- Data Science and Modeling
With AI modeling, CUs can predict members’ behavior. Past transactions can predict future financial decisions, and armed with that knowledge, CUs can do things like identify opportunities for cross-selling of products at just the right time. AI modeling can also pinpoint members who might be thinking about leaving the institution, allowing a CU precious time to reach out to those members before they exit. In order to deploy predictive modeling and be responsive to changing members’ needs, FIs need clean and categorized data. Period.
- Targeted, Automated Marketing
With the intelligence pulled from cleansed transaction data, an FI can identify members based on a wide variety of behaviors. Armed with that knowledge, they can build audiences and reach out to only those members who are interested in particular products the CU offers. Best of all, cleansed data can be used to power marketing automation campaigns by keeping audiences accurate, making relevant messaging to members an easier lift on existing in-house teams.
- Uncovering Hidden Opportunities within an Existing Member Base
For example, identifying the members who are running small businesses out of their consumer accounts. How big of an “opportunity” is it? It may come as a surprise to hear that 60 million Americans did freelance work last year, according to gig economy platform Upwork – that’s 39% of the U.S. workforce. Segmint (an Alkami company) client data, presented at last year’s FinXTech Conference, showed that 26% of consumer deposit accounts showed ongoing business transactions and payments. Examining data from FIs in five asset classes, it was also discovered that there were between two and three times the number of “hidden” business accounts than there were actual business accounts. That’s a huge opportunity for CUs to reach out to those members, create business accounts and process those transactions.
The bottom line is
Data insights used to inform growth strategies, product development, and member retention tactics are here to stay. FIs of all sizes are hungry for intelligence, and members are begging to be treated as the unique individuals they are. Financial institutions who don’t employ data insights for the sake of their business and account holders are likely to lose out.
Alkami Transaction Data Cleansing enables FIs to better understand transaction behavior and model spend patterns. It transforms complex transaction descriptions into simple merchant names, merchant categorizations, and logos to normalize and contextualize the data. Our library science methodology blends human and machine capabilities to provide the fastest and most accurate data enhancement available. Learn more by visiting Alkami.com.