Fair Lending: Using AI to democratize compliance
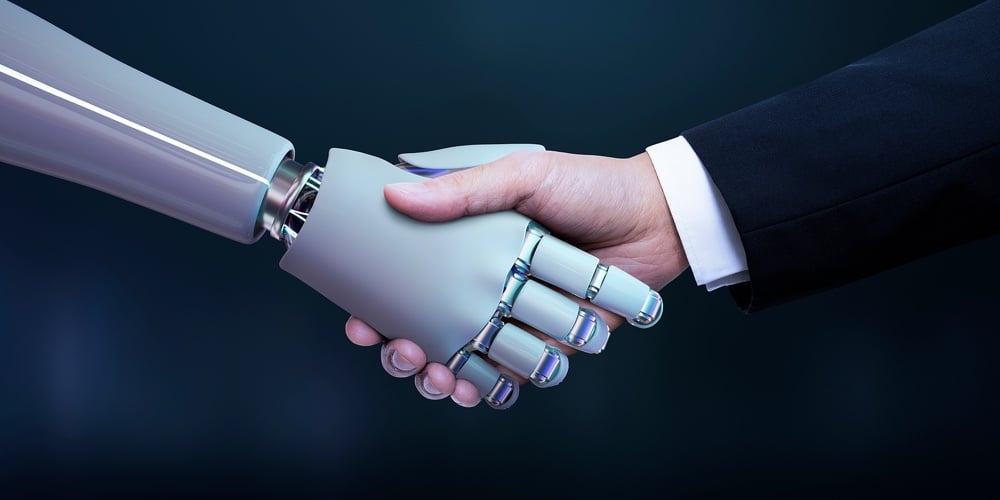
In its most recent advisory, the CFPB addressed a critical question – “When creditors make credit decisions based on complex algorithms that prevent creditors from accurately identifying the specific reasons for denying credit or taking other adverse actions, do these creditors need to comply with the Equal Credit Opportunity Act’s requirement to provide a statement of specific reasons to applicants against whom adverse action is taken?
The answer is an obvious ‘Yes’.
With the CFPB’s circular reminding everyone of adverse action notice requirements under the ECOA Act, some credit unions find themselves in a quandary when it comes to explaining their credit decisions, which is perceived to be difficult when they use state of the art decisioning algorithms. However, modern AI solutions have moved beyond mere aspects of explainability to enable fair lending, and have gone the extra mile to remove inherent biases that may arise in data based models.
Nonetheless, it is necessary to understand the CFPB’s guidance and how AI can effectively be a solution itself.
Looking back:
The use of algorithms in making lending decisions is not something novel or new. Credit risk assessment naturally requires getting your arms around as much relevant data as you can. A mix of models and algorithms have been the backbone of credit decisions for around 4 decades now, with credit analysts using financial statements, credit histories, and other data sources to estimate credit risk, set credit limits and recommend payment plans. With time, the datasets in question have become so voluminous that lenders had to move from manual methodologies to computational models for analysis of data using analytics.
Recent advancements in computational methods have introduced the “AI” element in lending processes to make credit risk assessments much more accurate. Artificial Intelligence and Machine Learning models leverage a diverse set of alternate data sources beyond bureau, and use historical training data to determine non-linear correlations between data points, and provide advanced predictive signals on member behavior and lending outcomes.
The unique proposition here is the ability of AI/ML models to analyze voluminous quantities of data, detect hitherto unknown correlations, and keep self-learning and adapting the models with little or no manual interventions.
The opportunity ahead with AI in lending:
AI enabled technologies have helped put the spotlight on the increasingly visible disparities in existing lending processes. A 2019 paper by Robert Bartlett & Co. helps quantify this disparity:
“Black and Latino applicants receive higher rejection rates of 61% compared to 48% for other races. In addition, they also suffer from higher ‘race premiums’ on interest rates, paying as much as 7.9 basis points more on mortgage interest rates. This difference has the impact of an additional annual race premium of over $756 million dollars per year in the United States.” (Source)
And these numbers present just one side of the picture: that of the creditworthy borrowers. They don’t highlight the disparity that occurs due to the presence of Credit Invisibles.
Credit Invisibles are borrowers who don’t have enough credit history or data on any of the three credit bureaus, making it difficult for them to be assessed for a credit decision. While almost 26 million Americans are completely credit invisible, another 19 million Americans have either very thin files or outdated files with a lack of recent credit history. And what’s more striking is the difference in numbers when it comes to races: About 15% of Blacks and Hispanics are credit invisible compared to roughly 10% of Whites and Asians. 13% percent of Blacks and 12% of Hispanics have unscorable credit records compared to about 7% of Whites and close to 8% of Asians. (Source)
So how does AI in lending hope to bridge this gap and mitigate discriminatory lending? The first part of it involves going beyond conventional sources of data for credit risk analysis. While alternate data has been used on some level in credit risk analysis, AI Models tend to focus on the relevant data, or inclusive data, that helps determine the creditworthiness of a member despite their low credit history. Foraying into a member’s FCRA compliant non-credit data, including for example, insurance payments, service payments, rents, property records, would help in accurately determining the credit worthiness of an applicant, even when there’s not enough credit information available. These data points not only help in credit risk analysis, but also help in customizing credit offerings as per the needs of members.
The second part involves leveraging AI to assess historical data for any inherent biases and evaluate new decisions through the lens of potential “Disparate Impact”
Disparate Impact Analysis (DIA) quantitatively measures the adverse treatment of protected classes. By analyzing member data, AI helps distinguish between inadvertent biases that may exist, and the actual factors that should be used to determine the credit worthiness of an applicant in a fair and consistent manner.
Using modern AI technology for compliant, fair and transparent lending:
Modern AI/ML underwriting tools have taken the extra step to inculcate the explainability and non-discriminatory aspects in their models. “At Scienaptic, we keep the regulatory aspects of Credit at the core of every decision and strongly support the CFPB’s circular guidance. It is quite apparent that every credit decision made by an AI platform needs to be explainable and free of bias”, comments Pankaj Kulshreshtha, CEO at Scienaptic AI, a leading AI based credit underwriting platform. “At the heart of building transparent, bias-free models are 3 core principles: transparent adverse action reasons, comprehensive disparate impact analysis, and well-elucidated documentation of model/decision parameters.”
- Adverse Action Reason (AAR) and letter
ECOA provides that a creditor must provide a statement of specific reasons in writing to applicants against whom adverse action is taken. Pursuant to Regulation B, a statement of reasons for adverse action taken “must be specific and indicate the principal reason(s) for the adverse action.” AI platforms have done just that, ensuring that irrespective of the algorithm used to assess the credit risk, the adverse credit decision always has “specific” and “principal” reasons associated with it. Modern AI is making the adverse action reason verbiage simple yet comprehensive and it ensures that reasons are “actionable” so that consumers can make appropriate adjustments to their future credit behavior. - Adequate testing for fair lending
ECOA makes it unlawful for any creditor to discriminate against any applicant, with respect to any aspect of a credit transaction, on the basis of race, color, religion, national origin, sex or marital status, age (provided the applicant has the capacity to contract), because all or part of the applicant’s income derives from any public assistance program, or because the applicant has in good faith exercised any right under the Consumer Credit Protection Act. Similarly, Fair Housing Act prohibits discrimination in residential real-estate related transactions.
In order to discount the bias due to historical training data, AI platforms have adopted a comprehensive disparate impact analysis, focusing on the ‘disparate impact’ of a credit decision. A disparate impact occurs when policies that appear to be neutral adversely impact protected groups. In order to combat the same, modern AI technologies focus on thoroughly reviewing policy attributes and ensuring no biases have crept into the model design, and conduct comprehensive tests to assess the discriminatory impact of credit decisions on certain protected classes, and take corrective actions. AI models rely on a feedback loop of continuous adaptive assessment to correct these inadvertent biases and come up with fair lending models. - Documentation of model/decision parameters
Model Risk Management and related documentation forms the center of any credit risk decisioning. The scope and nature of activities in this area have been ever evolving but the cornerstone lies in the Federal Reserve and Office of the Comptroller of the Currency (OCC) Supervisory Guidance on Model Risk Management ( SR11-7), which is intended for use by banking organizations and supervisors as they assess organizations’ management of model risk. This guidance applies as appropriate to all banking organizations supervised by the Federal Reserve, taking into account each organization’s size, nature, and complexity, as well as the extent and sophistication of its use of models
AI platforms follow the key principles laid out in the guidance and take into account each organization’s size, nature, and complexity, as well as the extent and sophistication of its use of models. AI platforms clearly lay out:
- Sound design, theory and logic underlying the model
- Rigorous assessment of data quality and relevance
- Adequate testing to ensure that various components of the model function as intended
- Robustness and stability of the model under varying conditions (Macro, Lender specific and others)
- Model Limitations and Assumptions
Conclusion:
Explainable AI models are bound to form the backbone of lending systems in the next 2 decades, as they become more and more indispensable for financial institutions in supporting their credit ecosystem. As such, while compliance and regulatory adherence is a necessity to ensure fair lending practices, AI tools should not be seen as an antithesis to fair lending, but rather an enabler. When the models in question are transparent and explainable, it would have a cascading effect empowering effect on regulatory bodies, credit unions, and members, resulting in better approval rates, lesser gap in approval numbers between different classes, lesser defaults, and overall, a healthy credit ecosystem. By leveraging an amalgamation of the right lending practices, the right models, and the right compliances, we will be able to achieve optimum member experience and enhanced credit flow at low risk.
Co-Author: Prateek Samantaray