What credit unions can learn from online dating
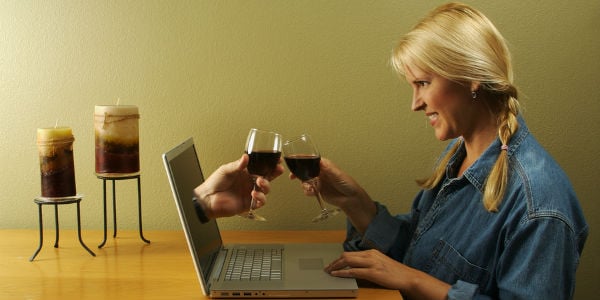
Online dating has grown significantly in recent years. According to a recent Pew Research Center study, one in five Americans aged 25-34 have used online research. This growth is attributable to the increasing acceptance of this method to meet people as well as the touted success from online dating services. And the key to building these successful “matches” comes from data.
Most online dating sites claim their proprietary compatibility algorithms are the magic behind delivering ideal candidates for successful relationships. Credit Unions are also looking to create successful relationships with their members. They know that the more the products and services a credit union member uses, they more loyal and profitable they are. The question, it seems, for credit unions is how build “compatibility algorithms” to create successful relationships? To answer this, lets take a closer look at the algorithms.
In online dating, two kinds of algorithms are used. They are matching and behavior algorithms. Matching algorithms work by collecting like responses to a survey. Then matching the responders who answered the survey question in the same way together. In a behavior algorithm, data is collected based off of action and intent responses to create trends and ultimately profiles. In online dating this can be a little trickier as what person may say they want may not reflect what they do (actions).
Bringing this back to credit unions, matching algorithms can be used slightly differently. Let’s look at successful relationships within the organization. In credit unions, a successful relationship can be defined as a new auto loan. To understand why the member opened the auto loan, may tell us why other, like members may open auto loans as well. To gather more information, lean into the core processor/MCIF where you can find out a plethora of information about the members who recently opened auto loans. You and find out how old the members are, where they live, how close they are to the branch/atm, what other products and services they use as well as if they use online banking (and mobile) as well as social media. You can also see their income and credit score and what method they chose to complete the loan application (online or offline.)
All of this data will create a “success” profile for you. You will be able to see the auto loan holders in terms of those who have successfully engaged with you. How do you match this data to new auto loans? Simply run a report of all your members who fit the success profile but who do NOT have an auto loan with you.
Of these prospects, see if they have auto loans at another lender. Determine how you can acquire these loans. If they do not have an auto loan, pre-approve them, send them information showing them how much the auto loan with your organization is better for them and their wallet. And of course make the loan application channel as simple as possible to apply, approve and get funded.
In regards to using behavior algorithms, these can be tricky for credit unions. Think about how many times you have surveyed members and they respond opposite of what they actually do. This being said, try the matching algorithm first and consider monitoring online member sentiment to evaluate trends and build a case study to use in the future.
Happy data digging & translation– I wish you much success!