Predictive models take the sting out of collections
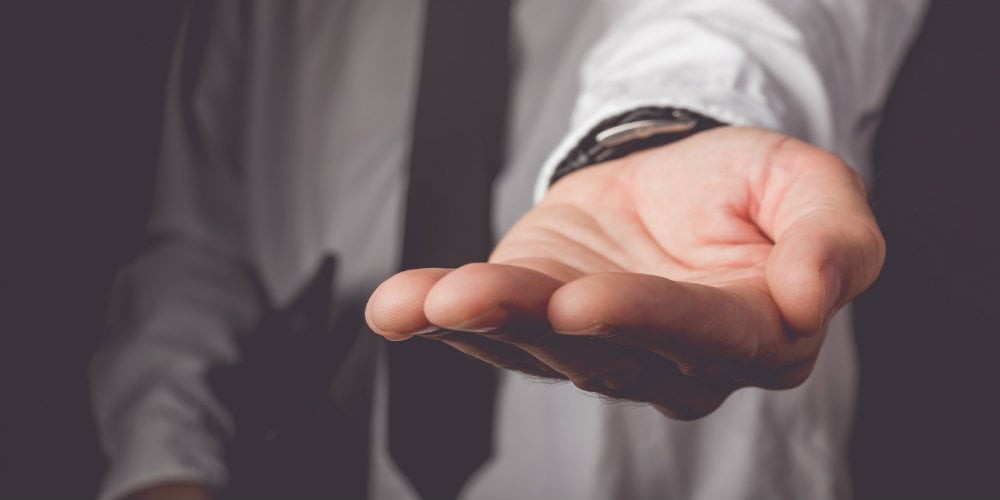
Collections is a touchy subject for people-centric credit unions. The human aspect to requesting repayment from already struggling members is difficult enough. Pile on the financial, regulatory and operations components and it becomes one of the most cringe-worthy aspects to running a sustainable financial services organization.
To ease some of the pain associated with collections, credit unions are relying on the foretelling power of data analytics. Predictive models deployed by credit unions can help in three very significant ways:
Selecting the Accounts
Collecting on every delinquent loan account is far from plausible, especially for credit unions with medium to large loan portfolios. Such an undertaking requires heavy financial and human resource investment, far outweighing the likely returns. That’s why most credit unions choose to pursue repayment on only a select number of accounts. But relying on age-old conventions, even instinct, for delinquent account segmentation is just as inefficient and costly as going after them all. What’s more, this kind of strategy rarely yields healthy returns.
A good collections strategy targets those accounts with a combination of likelihood to repay and severity of loss. A data-driven approach to selecting only those accounts can solve many of the pervasive problems that exist within credit union collection efforts. Yet, segmenting delinquent accounts solely on credit scores and current delinquency stage is not enough. Collection analytics, a data-driven predictive method, is based on much more than traditional data points, and it identifies much more than a set of accounts.
Choosing the Channels
Collections analytics not only enables identification of accounts with the highest propensity for repayment, it also helps collections teams understand the best channels through which to engage with a particular member.
Homegrown collections teams often attempt to inspire repayment with a variety of communication – from emails and texts to manual or auto-dialed calls. Online and mobile efforts, too, are adding to the growing list of choices for how to contact delinquent members. Over 180 days (the length of time most credit unions wait before turning an account over to an agency or debt buyer), those communication efforts add up. With substantial costs to administer them, collections teams are often left wondering if their efforts are actually costing the credit union more than they are saving.
With the guiding light offered by collection analytics, however, credit unions can laser target those communication efforts to the right accounts, early and consistently. Additionally, tracking member response to the outreach is key. It adds another dimension to the predictability of willingness to repay. Armed with this information, the rate of recovery increases, boosting efficiency and lowering costs. Another highly important benefit is a reduction in the number of credit union member accounts turned over to less people-centric organizations, such as debt buyers and collections agencies.
Deciding When to Act
By aggregating structured and unstructured data into a single repository, data scientists and analytics experts employed by credit unions can spot patterns among delinquent accounts. This trend spotting helps collections teams target the right member through the right channel, and importantly, at the right time.
During early stages of delinquency, members may not require multiple calls. They may simply need a reminder of the outstanding balance. Some may even self-cure with no intervention or communication. Led by data analytics, credit unions can shift some call center staff from early- to late-stage recovery efforts. Doing so will deliver better returns and reduce costs, without falling below the optimal collection rate for early-stage delinquencies.
Collection analytics can also help increase the dollars recovered and save litigation charges for credit unions at later stages of debt collection. Data scientists can also advise credit unions on the best course of action for accounts 180 days past due. Based on various analytics parameters, they can determine if and when a lawyer should be retained, an account should be handed over to an agency or sold to a debt buyer.
Importantly, predictive modelling has been shown to help members proactively reduce their payments before going delinquent or charging off. Monitoring for financial stress among members, for example, can trigger outgoing text communication inspiring repayment more quickly. Clicking on a link in that text prompts the member to accept the reduction of their monthly payment up to a modelled amount.
For many credit unions, preservation of the member relationship – even when that member is costing the cooperative essential revenue – is paramount. Indeed, there is nothing that inspires loyalty more than seeing someone through tough times. Two-way dialogue during financial stress is a great first step. However, the hands-on, caring approach will not always result in a positive outcome for the cooperative or for the member. Data-centric, predictive analytics can soften the pain associated with debt recovery by giving the collecting credit union important information ahead of the recovery efforts. The more a credit union knows about its member, the less invasive it can be to get that individual back on the right track.